Machine Learning and Log Analysis: Uncovering Hidden Needles in Your Haystack
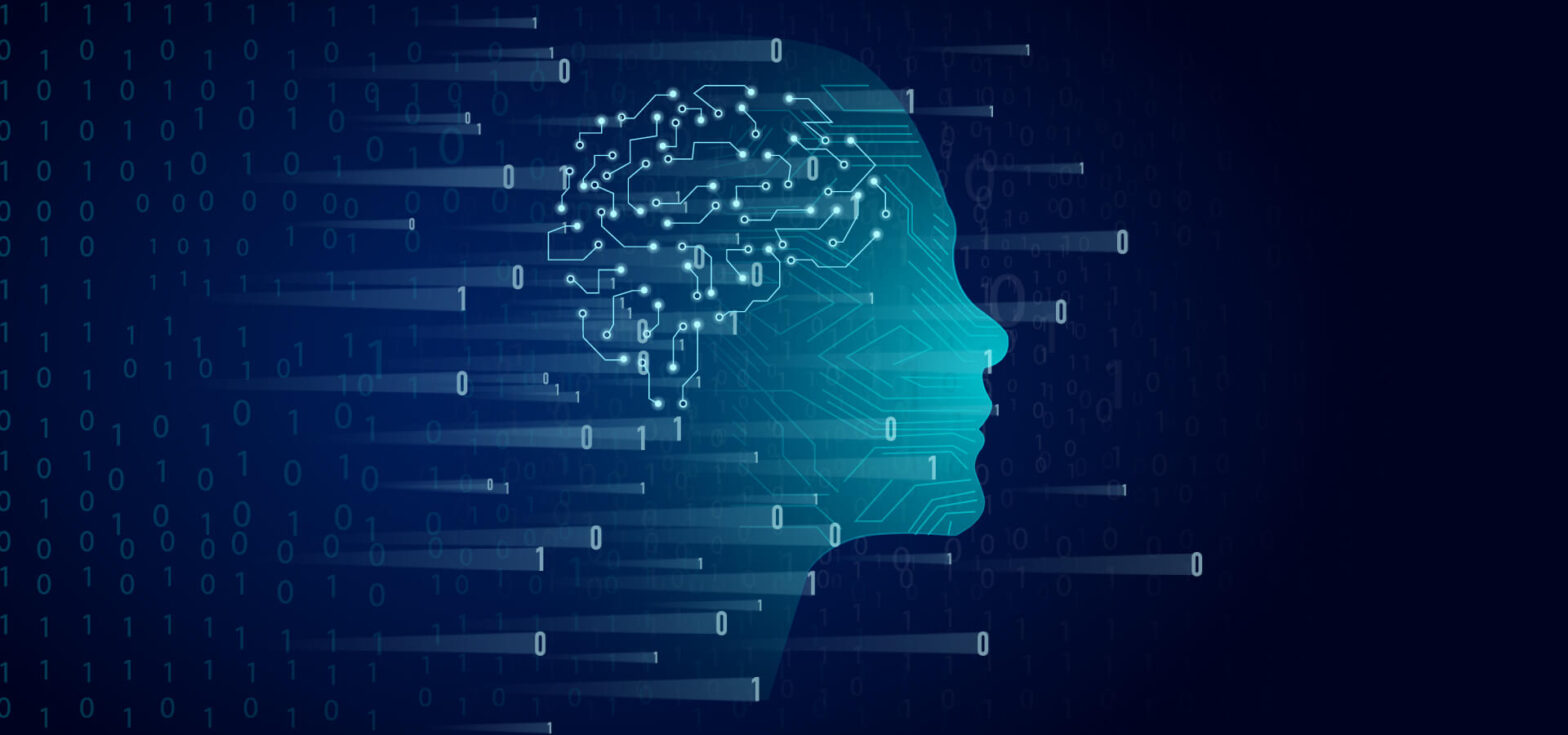
Manual threat detection methods can’t keep up with the evolving tactics of cybercriminals. Machine learning (ML) can analyze large volumes of data quickly and accurately to detect potential threats. In this blog, we explore how ML can transform cybersecurity and keep our systems safe.
Introduction
The world of cybersecurity can be complex and overwhelming. It’s like looking for a needle in a haystack of log data, where potential threats are hidden amongst the overwhelming volume and complexity of data. Every day, organizations face the challenge of sorting through terabytes of logs, hoping to find clues to identify malicious threats or anomalies. But this manual process is time-consuming, prone to human error, and struggles to keep up with the constantly evolving attack methods used by cybercriminals. Traditional threat detection methods often fall short of catching the sophisticated tactics used by malicious actors. Machine learning (ML) is a dynamic and capable tool in the digital world that can help efficiently and accurately analyze vast amounts of log data, uncovering threats that might otherwise remain undetected. This technology can potentially transform how we approach cybersecurity and keep our systems safe.
Haystack of Logs
The sheer volume, velocity, and variety of log data generated by modern systems is expanding rapidly and is nothing short of staggering. Terabytes of logs are produced every second, capturing all the actions, events, and transactions happening across the digital ecosystem. This escalating growth poses a major challenge for traditional security tools, which can struggle to keep up with the scale and complexity of the data deluge.
In the age of information, it’s easy to overlook valuable security insights amidst the abundance of available data. More often than not, crucial indicators of malicious activity are hidden among the haystack of logs, making real-time threat detection feel like searching for a needle in a stack of needles. The sheer volume of benign data can easily lead to overlooking critical alerts.
Compounding this challenge, security teams also deal with an overwhelming number of false alarms triggered by traditional methods. These false positives create confusion and waste valuable time and resources. Moreover, they divert attention from real threats, which remain hidden in the shadows.
Analyzing huge amounts of data manually is a challenging task, which would require a large team of analysts and an indefinite amount of time. Even with a dedicated workforce, the sheer size of the data makes manual inspection impractical, if not impossible. This leaves organizations vulnerable to undetected security threats that may be hidden within the data.
Furthermore, traditional rule-based security systems are no longer effective in keeping up with the constantly evolving tactics of threat actors. These systems rely on static rule sets that can’t adapt quickly enough to counter emerging attack vectors. As the threat landscape continues to change, the limitations of rule-based systems become more apparent, highlighting the need for a more agile and proactive approach to threat detection.
Machine Learning Comes to the Rescue
The challenge of detecting hidden threats from a vast amount of logs can be overwhelming, but machine learning offers a promising solution. Unlike humans, machine learning algorithms can analyze large volumes of data with lightning speed and unwavering accuracy. Using advanced statistical techniques, ML models can sift through logs, detecting subtle anomalies and patterns that often elude the human eye. This makes machine learning a valuable tool that helps find that needle in the haystack.
In the field of cybersecurity, machine learning (ML) algorithms are utilized in advanced platforms equipped with a range of techniques designed for threat detection. Anomaly detection algorithms identify anomalies from normal behavior, warning security teams of possible malicious activity. Supervised learning models trained on labeled datasets can accurately identify known threats, while unsupervised learning algorithms reveal new threats that are hidden in the background without the requirement of predefined labels.
Imagine a situation where a cybersecurity platform powered by machine learning detects a malware infection in an organization’s network. By analyzing log data from different endpoints and network devices, the platform’s anomaly detection algorithms can identify unusual patterns of file access and execution that may indicate a potential malware outbreak. At the same time, supervised learning models trained on historical malware samples can quickly classify the threat, allowing security teams to take instant action to contain and eliminate the infection.
In another scenario, unsupervised learning algorithms can uncover a series of unauthorized access attempts across multiple user accounts, signaling a potential data breach in progress. With these insights, security teams can act quickly to mitigate the breach and protect sensitive information from being compromised. In both cases, machine learning proves to be an asset in the ongoing fight against cyber threats. By transforming the haystack of logs into actionable intelligence, ML helps security teams stay a step ahead of cybercriminals.
Your Dynamic Threat Defense Platform
Welcome to the cutting-edge world of modern cybersecurity defense with NewEvol, our Dynamic Threat Defense Platform. Our all-in-one cybersecurity platform uses machine learning for advanced threat detection and response. Harnessing the unparalleled capabilities of ML, it navigates through the haystack of logs with accuracy and agility, making it an innovative solution for any organization concerned about cybersecurity.
With the advanced log aggregation capability of the Data Lake solution, NewEvol consolidates logs from different sources into a central repository for comprehensive analysis. It uses sophisticated anomaly detection algorithms to sift through vast volumes of log data, quickly identifying any variations from normal behavior that could indicate potential threats. Each anomaly is thoroughly evaluated and assigned a threat score, giving security teams actionable insights into the severity and urgency of the threat.
Our DTD platform is equipped with an automated incident response capability that enables organizations to respond to security threats in real time with remarkable speed and efficiency. This feature automates response actions based on predefined rules and policies, which helps reduce response times and mitigate the impact of security incidents before they escalate into severe breaches.
Our Dynamic Threat Defense Platform has been tested and proven in the real world, helping organizations across various industries detect and respond to threats more efficiently. It can detect sophisticated malware infections, stop unauthorized access attempts, and mitigate data breaches, ultimately safeguarding sensitive assets and preserving the integrity of organizational networks.
A client sought a solution to monitor various smart devices in a smart city, with a wide range of devices placed in public places. Compliance requirements set by the government also had to be met. We deployed a Security Information and Event Management (SIEM) system with analytics and threat intelligence capabilities to monitor the smart city’s end-to-end environment, including custom alerts, dashboards, and reports. Machine learning algorithms detected anomalies in large volumes of network traffic on public devices, and threat intelligence capabilities kept the analyst updated with the latest threat feeds.
With NewEvol, enterprises can stay one step ahead of cyber threats, transforming the haystack of logs into a powerful tool for proactive defense in today’s ever-evolving threat landscape.
Conclusion
Amidst the overwhelming volume and complexity of logs, machine learning offers unprecedented speed, accuracy, and agility in threat detection. With our Dynamic Threat Defense Platform, organizations can consolidate logs, detect anomalies, and automate response actions to stay ahead of threat vectors and protect sensitive assets. Join us in redefining the future of cybersecurity and Book a Demo today.